Abstract
The utilization of omic modalities for understanding the molecular basis of common diseases and traits is increasingly prevalent. Although multi-omic traits are useful for cost-effective and powerful analyses, studies that lack multi-omic data can still predict these traits genetically.
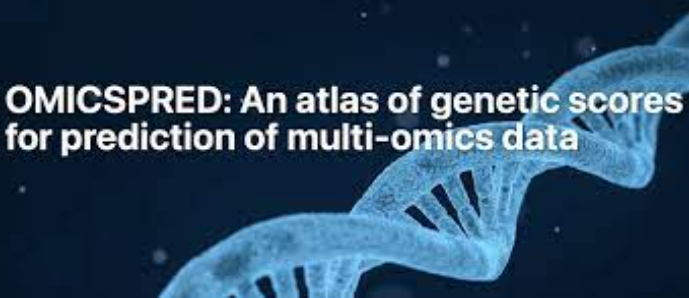
In this study, we examine the INTERVAL cohort (n=50,000 participants), which has extensive multi-omic data for plasma proteomics (SomaScan, n=3,175; Olink, n=4,822), plasma metabolomics (Metabolon HD4, n=8,153), serum metabolomics (Nightingale, n=37,359), and whole-blood Illumina RNA sequencing (n=4,136). We use machine learning to train genetic scores for 17,227 molecular traits, including 10,521 that reach Bonferroni-adjusted significance.
To evaluate the performance of these genetic scores, we conduct external validation across cohorts of individuals with European, Asian, and African American ancestries.
Additionally, we demonstrate the usefulness of these multi-omic genetic scores by identifying disease associations using a phenome-wide scan and quantifying the genetic control of biological pathways.
We highlight several biological insights, such as the association between JAK-STAT signaling and coronary atherosclerosis.
Lastly, we develop a portal (https://www.omicspred.org/) to provide public access to all genetic scores and validation results and to serve as a platform for future improvements to multi-omic genetic scores.
Data availability
The genetic-score models that were trained in this study and the GWAS summary statistics utilized to create them can be accessed publicly through the OmicsPred portal (https://www.omicspred.org/). The accession codes for the genetic scores range from OPGS000001 to OPGS017227.
Researchers who meet the necessary qualifications may obtain access to the INTERVAL study data discussed in this paper by contacting [email protected]. Additional information regarding the data access policy is available at http://www.donorhealth-btru.nihr.ac.uk/project/bioresource.
Code availability
You can access the original codes used for training genetic scores with INTERVAL data, as well as for internally validating these scores and evaluating the performance of various genetic score construction methods, by visiting https://github.com/xuyu-cam/atlas_genetic_scores_omic_traits.